The physical characteristics of the solar wind, captured through in-situ measurements by satellite sensors and magnetometers, can be employed to more accurately determine the solar wind's coronal origins and more accurately predict the data for the future Heliophysics phenomena.
The accurate classification of solar wind’s coronal origins directly impacts space weather forecasting, protecting and designing space missions, developing early warning systems for space and weather events, and a holistic understanding of our solar system.
Our project aims to explore:
1. How dimensionality reduction techniques can be used to understand the relationships between in-situ measurements and various coronal origin classification approaches.
2. Clustering methods to objectively cluster the low-dimensional representation of the solar wind data to better understand the potential differences among the categories caused by the different coronal origins.
3. How ensemble and deep learning methods are being used to forecast sunspot numbers, enabling scientists to theorize about the future impact of solar activity and space weather.
You can explore the code for this project on GitHub.
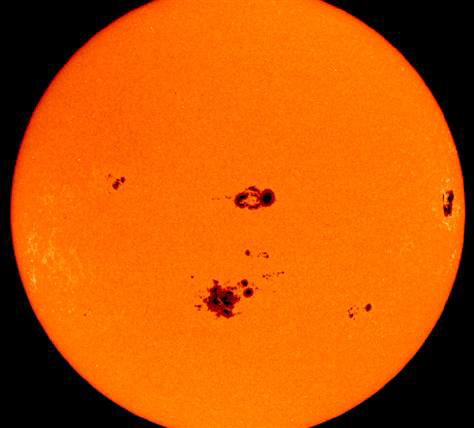
Dark regions on the Sun's surface are known as sunspots, cooler-temperature regions caused by strong, local magnetic activity. Photo Courtesy of NASA.
Project Findings
Understanding the in-situ Solar Wind Properties with Machine Learning
Team Interstellar - Sarah Amiraslani, Andria Lesane, & Nikola Acin (April 2024)